[Thesis defense] 25/04/2024 - Imen BEN-AMOR, "Deep modelling based on the notion of voice attributes for explicable speaker recognition: application to the forensic domain"(LIA)
Title of the thesis
"Deep modelling based on the notion of voice attributes for explicable speaker recognition: application to the forensic domain".
Date and place
Oral defense scheduled on Thursday 25 April 2024 at 2pm
Location: 339 Chem. des Meinajaries, CERI, 84000 Avignon
Room: Amphi ADA
Discipline
Computer Science
Laboratory
UPR 4128 LIA - Avignon Computing Laboratory
Framing
- Mr Jean-François BONASTRE
Composition of the jury
Mr Jean-François BONASTRE | Avignon University | Thesis supervisor |
Mr Tomi KINNUNEN | University of Eastern Finland | Rapporteur |
Mr Alessandro VINCIARELLI | University of Glasgow | Rapporteur |
Ms Tanja SCHULTZ | University Bremen | Examiner |
Didier MEUWLY | University of Twente | Examiner |
Ms Corinne FREDOUILLE | Avignon University | Examiner |
Summary of the thesis
Automatic Speaker Recognition (ASR) has been integrated into a variety of applications, from access security to forensic identification. Its aim is to automatically determine whether two speech samples come from the same speaker. RAL systems are mainly based on complex neural networks (DNNs) and present their results as a single value. Despite their high performance, they are unable to provide information about the nature of the speech representations used, their encoding and their influence on decision-making. This lack of transparency poses significant challenges in addressing ethical and legal concerns, particularly in high-risk applications such as forensic voice matching. This thesis introduces a three-stage approach based on deep learning, designed to provide interpretable and explainable RAL results.
In the first step, we represent a vocal excerpt by the presence or absence of a set of vocal attributes, shared between groups of speakers and selected to be discriminating from the speaker's point of view. This information is encoded by a binary vector where a coefficient equal to 1 indicates the presence of the corresponding attribute in the speech extract and 0 its absence. This representation provides interpretability, while offering a level of performance close to that of RAL's state-of-the-art systems (SOTA).
The second stage involves the explicit calculation of the RAL score, represented here by a likelihood ratio (LR). For this, we propose a method called BA-LR, which breaks down the calculation process into sub-processes, each dedicated to an attribute. An attribute LR is estimated for each attribute using only the presence or absence of the attribute and its description, defined by three explicit behavioural parameters. The final LR is calculated as the product of the attribute LRs, assuming their independence. This estimation allows a transparent calculation of the LR, combined with detailed explanations of the contribution of each attribute to the final LR value, which can help users, such as judges, in their decision-making.
The third stage is dedicated to discovering the nature of the attributes. We propose an automatic description of the attributes into acoustic, phonetic and phoneme information using different explicability methods. The explanations obtained provide a better understanding of the voice attributes used in RAL and offer new perspectives for phoneticians. To validate the effectiveness of our approach in forensic science, we evaluated it using a database specific to this field. To do this, we defined a calibration approach adapted to the field. The results demonstrate the robustness and generalisability of BA-LR in a forensic context. The various contributions of this thesis open up a new perspective in terms of explicability in RAL, by proposing to accompany the inference, the LR, with the explanations necessary for transparent decision-making, with a level of performance comparable to SOTA systems. In forensic science, our approach seems promising, making it easier for experts to understand the elements of a decision and for the court to take them into account. It also offers phoneticians a tool for better understanding speech information. However, these encouraging results need to be developed further with a variety of use cases before being applied in real forensic contexts, while respecting the 'duty of care' specific to this field.
Keywords
Speaker recognition, Neural networks, Explicability, Interpretability, Voice attributes, Forensics
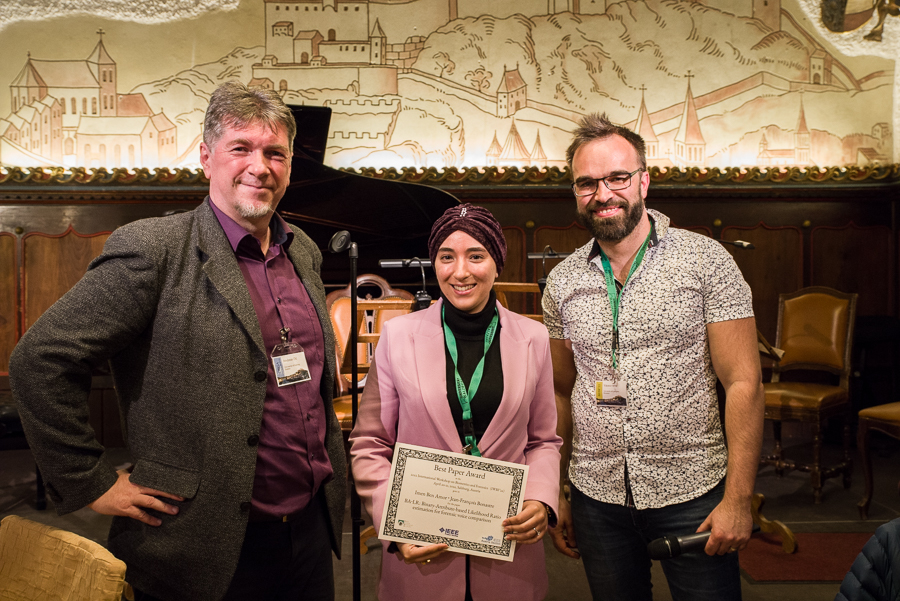
Mis à jour le 23 April 2024