[PhD defence] 13/12/2024 - Tiancheng YANG: "Phenotyping the density of small grain cereal plants at the early stages using optical sensors". (UMR EMMAH)
Tiancheng Yang will submit his thesis on 13 December 2024 on the subject of "Phenotyping the density of small grain cereal plants at the early stages using optical sensors".
Date and place
Oral defense scheduled on Friday 13 December 2024 at 9.00 am
Location: Address: Domaine Saint-Paul - Site Agroparc, 228 Rte de l'Aérodrome CS 40509, 84914 Avignon Cedex 9
Building : The heart of the centre
Postcode: 84914
City: Avignon
Room: Provence Amphitheatre
Discipline
Agricultural sciences
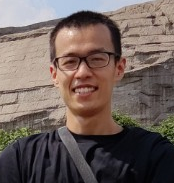
Laboratory
UMR 1114 EMMAH - Mediterranean Environment and Modelling of Agrohydrosystems
Composition of the jury
Mr Sylvain JAY | INRAE | Thesis supervisor |
Mr Wei GUO | Graduate School of Agriculture and Life Sciences, University of Tokyo | Rapporteur |
Mr David ROUSSEAU | ImHorPhen team, Angers University | Rapporteur |
Mr Shouyang LIU | Nanjing Agricultural University | Examiner |
Ms Katia BEAUCHENE | ARVALIS | Examiner |
Mr Andreas HUND | ETH Zurich, Institute of Agricultural Sciences | Examiner |
Summary
Small grain cereal plant density is a key trait in plant phenotyping, crucial for both plant breeding and precision agriculture. The usual method based on counting plants is laborious and low throughput, making the development of an automatic, high-throughput method based on optical data highly desirable. However, such a method must meet several challenges that have not been fully studied in the literature: it must manage occlusions, have sufficient throughput, estimate the soil surface and be generalisable to other experiments. In this thesis, we developed several methods for estimating plant density using spectral reflectance or high-resolution images as input data, and provided answers to these four challenges. This work is based on a large database collected over the last three years, including several sites, years, growth stages, varieties (and even species), viewing angles and optical sensors.
In Chapter 2, we showed that the green fraction can be used as a proxy for plant density, but this requires calibrating a model for each site, growth stage and variety. An error of 30% was obtained using the green fraction estimated from spectral reflectance, while an error of 22% was obtained using the green fraction derived from RGB images with a spatial resolution finer than 1 mm/pixel.
In Chapter 3, we explored a two-step process based on RGB images: a plant counting step based on the P2PNet detection model, followed by a soil surface estimation step based on the P2PNet model outputs. This method made it possible to estimate plant density on independent datasets with an error of around 30 %, which is mainly due to the plant detection step (10 % error for soil surface estimation).
In Chapter 4, we proposed to detect leaf tips rather than plants in the images, in order to better address the challenges related to occlusions and generalisation capability. The leaf density obtained by counting the leaf tips present in an area of known size was used to estimate the plant density by inversion of a dynamic crop model. An estimation error of 10 % was obtained on the same independent data sets using two observations made at appropriate growth stages.
Finally, in Chapter 5 we propose several avenues of work, one of which is to combine the results obtained in this thesis to design two methods that meet the four challenges mentioned above. Other avenues include improving annotation rules for deep learning detection, and applying the method presented in Chapter 4 to estimate phyllochrony.
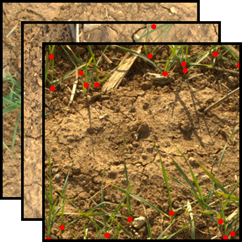
Keywords : Crop phenotyping, plant density, small grain cereals
Updated le 9 December 2024